Navigating Risks In Market Research : Making Informed Investment Decisions In Private Equity
Exploring the Use of Synthetic Data in Market Research for Private Equity Firms
In the realm of market research, synthetic data is gaining traction due to its potential to enhance data quality, protect privacy, and reduce costs. However, its application is not without risks. This blog post discusses the use of synthetic data for a specific use case: a private equity firm conducting expert calls in the social care, dementia care, and primary care spaces to understand the software pricing of rostering, care planning, and compliance solutions.
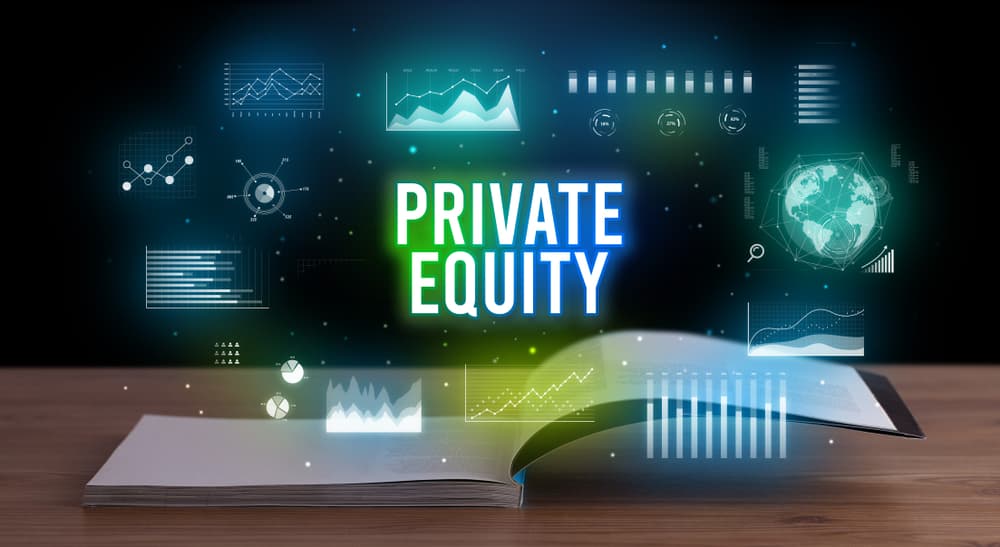
The Use Case: Private Equity Firm in Healthcare Software Pricing
Private equity firms often seek detailed market insights before making investment decisions. The use case today is a PE firm that wants to gather information on the pricing of software solutions in social care, dementia care, and primary care. These solutions include rostering, care planning, and compliance software. Traditional market research methods can be time-consuming and expensive, and this is where synthetic data can play a role.
Benefits of Synthetic Data
- Enhanced Data Quality: Synthetic data can augment real datasets, especially when the available data is limited or imbalanced. This can improve the robustness and accuracy of market research models.
- Privacy Protection: Synthetic data helps protect sensitive information, making it easier to comply with data privacy regulations such as GDPR. For example, when conducting market research involving sensitive health data, synthetic data ensures that no real patient information is used, thereby protecting individual privacy.
- Cost Efficiency: Instead of conducting numerous expensive expert interviews, synthetic data can simulate expert responses based on existing data patterns, saving both time and money. Needless to say, generating synthetic data can be more cost-effective than collecting large volumes of real-world data.
Risks of Using Synthetic Data
Despite these benefits, there are significant risks associated with the use of synthetic data:
- Data Authenticity and Reliability: If synthetic data fails to account for regional pricing differences in software, the analysis may provide a skewed view of the market. If the synthetic data does not capture the nuanced pricing strategies of software vendors in the healthcare sector, the firm might make misguided investment decisions. Synthetic data could therefore not accurately reflect real-world scenarios, leading to potential inaccuracies in insights.
- Bias in Data Generation: In our use case, we want to have a more balanced training dataset. If the training data for generating synthetic care planning software prices only includes data from high-income regions, the synthetic data may overestimate prices for lower-income regions. This means that the algorithms generating the synthetic data are based on biased training data (which leads to biased synthetic data). This could skew the analysis and lead to incorrect conclusions about software pricing and market dynamics.
- Limited Generalizability: Along the same lines, synthetic data will fail to generalize actual market conditions well if the original data or the model used for data generation does not capture all relevant variables.
- Validation Challenges: Without a reliable benchmark, it is difficult to confirm that synthetic data accurately represents real-world conditions. Validating the accuracy and utility of synthetic data is challenging without access to high-quality real-world data for comparison. This reiterates the need to ensure balance in the training dataset.
- Legal and Ethical Concerns: While synthetic data mitigates some privacy issues, it does not eliminate all legal and ethical concerns. Firms must ensure that the synthetic data generation process complies with all relevant regulations. In healthcare, for example, using synthetic data without proper consent or transparency can still raise ethical concerns and legal challenges.
- Complexity in Data Integration: Regardless of what industry we are managing compliance for, it is crucial to ensure that synthetic rostering data accurately aligns with real-world compliance standards. This requires sophisticated data integration methods to prevent inconsistencies. The process of integrating synthetic data with actual data is complex and necessitates advanced techniques to ensure that the combined dataset remains coherent and functional.
Mitigation Strategies
To mitigate these risks, private equity firms can adopt the following strategies:
- Thorough Validation: Implement robust validation techniques to compare synthetic data against real-world data to ensure its accuracy and relevance. You need to cross-validate with real-world data!
- Bias Mitigation: Use diverse and unbiased training datasets to generate synthetic data and employ bias detection algorithms to identify and correct biases. The way to do this is to include a wide range of data points from different regions and income levels to help reduce bias in synthetic software pricing data.
- Comprehensive Integration: Develop sophisticated data integration methods to ensure synthetic and real-world data are combined effectively.
- Compliance with Regulations: Stay updated with data privacy regulations and ensure the synthetic data generation process complies with all legal requirements. Regularly reviewing and updating data generation practices can help maintain compliance with GDPR and other regulations.
- Investing in Expertise: Invest in the necessary technology and expertise in data science and machine learning to generate and utilize high-quality synthetic data.
By addressing the potential risks and implementing effective mitigation strategies, private equity firms can leverage synthetic data to derive valuable market insights. This is beneficial when exploring software pricing in the healthcare sector, allowing firms to gain comprehensive and accurate data without the high costs and privacy concerns associated with traditional methods.
Thank you for taking the time to delve into this important topic with us. Your interest in advancing market research through innovative solutions is truly appreciated.
Sources
Thagaard, Knut, et al. "Synthesizing Privacy-Preserving Data Using Generative Adversarial Networks." IEEE Access, vol. 7, 2019, pp. 30375-30384.
Zhao, Hongwei, et al. "Bias and Fairness in Artificial Intelligence Systems." Journal of the American Medical Informatics Association, vol. 28, no. 6, 2021, pp. 1210-1216.
Rubin, Donald B. "Statistical Issues in the Use of Synthetic Data for Confidentiality Protection." Journal of Official Statistics, vol. 9, no. 2, 1993, pp. 461-468.
Gaboardi, Marco, et al. "Privacy-Preserving Data Analysis with Synthetic Data." Journal of Privacy and Confidentiality, vol. 7, no. 1, 2017, pp. 68-98.